AI Personality Study
Do the personalities of large language models, including their tone, style, and values, change depending on which language we prompt them in—and should we care?- Time
- Location
- Cambridge, USA & Virtual
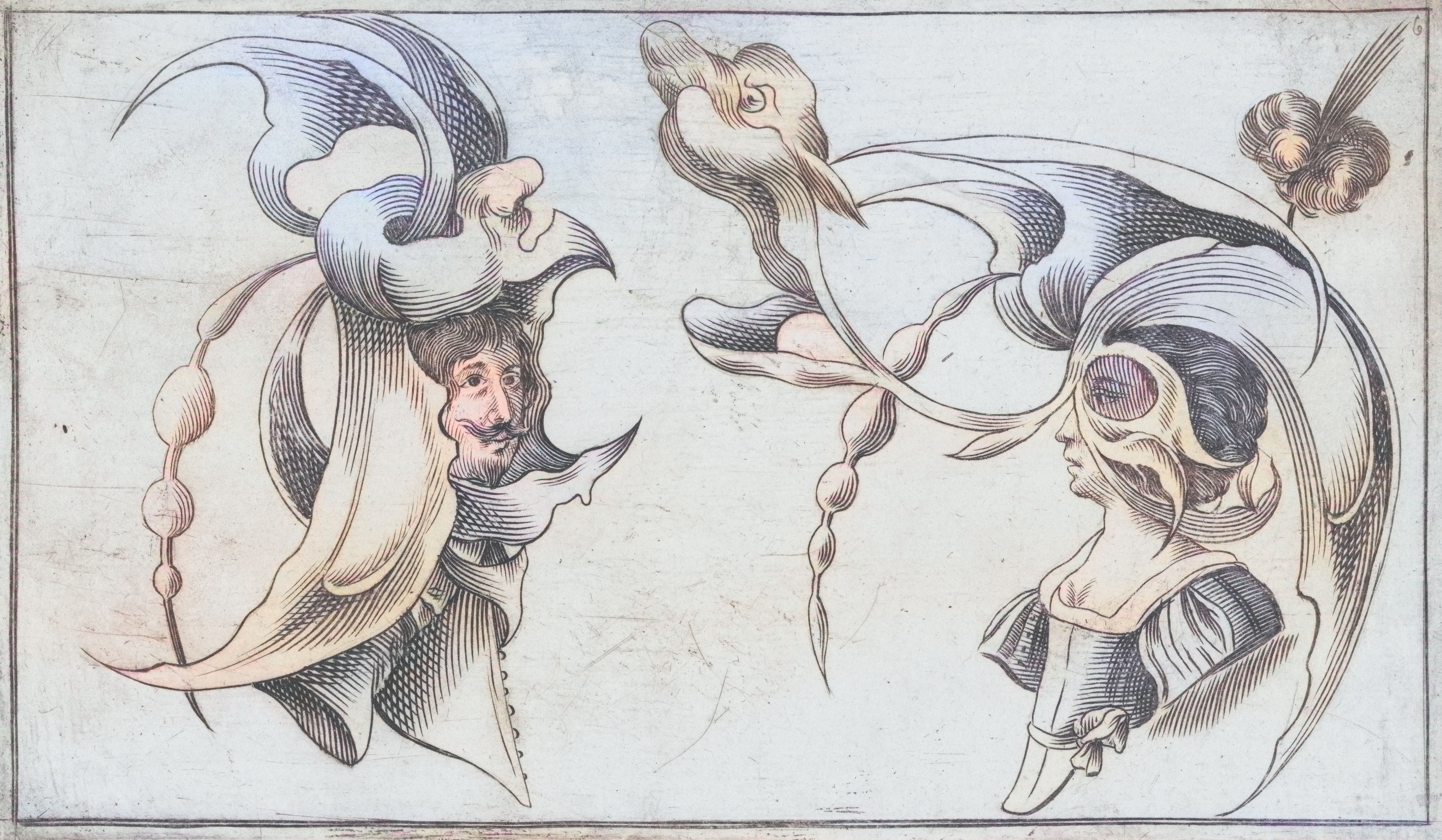
A new feature advertised alongside all of the latest large language models (LLMs) are “multilingual” capabilities. OpenAI states that ChatGPT can respond in over 50 languages, while Meta and Anthropic recently expanded their models to support 7 non-English languages. Beneath these claims lies a more complex reality: LLMs are inherently shaped by the biases of their training data and the humans involved in the fine-tuning process. Recent investigations have critiqued how LLMs perpetuate stereotypes and spread misinformation, especially towards racial and cultural groups. When it comes to language, no text is truly neutral—style, tone, and word choice reflect cultural contexts, assumptions, and values. This applies to LLM outputs as well, which invariably embody “personalities” that can shift when the same model is prompted in different languages, raising questions about consistency, fairness, and cultural representation.
In one of the first studies of its kind, metaLAB (at) Harvard is launching a comparative investigation of the personalities and linguistic styles of LLMs across different languages, with a particular eye to differences between high- and low-resource languages; in this context that refers to how much of the language is available in the form of digital text on the internet for training data for these models. Through a series of research workshops, multi-lingual participants will evaluate the outputs of LLMs by prompting them in different languages, analyzing not only what the models respond with but how they say it. The study will document these findings, offering insights into the linguistic styles and cultural subtexts that emerge across languages.
Alongside empirical documentation, this project explores a slew of normative questions around AI, language, and multiculturalism. Should we embrace variance, thinking that it reflects an accurate cultural personality associated with a given language? Or should we attempt to correct for inconsistencies, which may simply result from inadequate training data for low-resource languages? As AI-powered chatbots become increasingly important tools for education and personalized learning, it is crucial to evaluate the potential unintended harms of stylistic variance. For example, are low-resource language users harmed by personality variance, picking up an informal education in how to speak and write from “teachers” who aren’t monitoring the effects of their style? Or does an attempt to correct for differences amount to cultural homogenization, setting English tonal standards as the default? Who determines the answers to these questions, and who bears the responsibility of designing – and regulating – these models, are key inquiries of this project.
This project is funded by Harvard University’s William F. Milton Fund Generative AI Grant.